Big Data Analytics: A Literature Review Paper
This paper presents a comprehensive literature review on the topic of Big Data Analytics. Big data has emerged as a significant phenomenon in recent years, with organizations harnessing its potential to extract valuable insights and drive informed decision-making.
This review synthesizes the existing body of research, exploring key concepts, techniques, challenges, and applications of Big Data Analytics. The paper highlights the current state of knowledge, identifies research gaps, and offers suggestions for future directions in this rapidly evolving field.
Big Data Analytics: Definition and Context
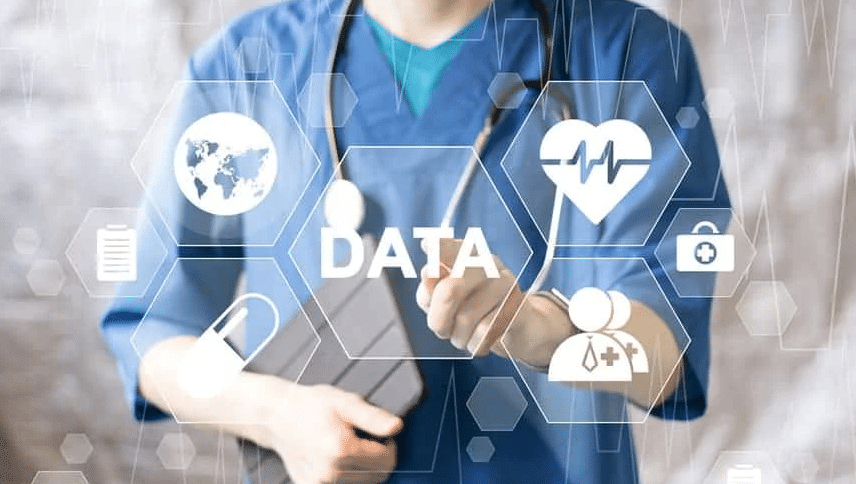
This section establishes a clear understanding of Big Data Analytics by defining its scope and contextualizing it within the broader field of data analytics. It outlines the unique characteristics and challenges associated with big data and emphasizes the need for specific capabilities to effectively analyze and derive insights from large and complex datasets.
Data Collection Capabilities
This section explores the capabilities related to data collection in the context of Big Data Analytics. It discusses techniques for data acquisition, data integration, data preprocessing, and data quality assurance. The section highlights the importance of comprehensive and accurate data collection to ensure the reliability and relevance of subsequent analyses.
Data Management Capabilities
The focus of this section is on the capabilities related to data management in Big Data Analytics. It covers topics such as data storage, data organization, data integration, and data governance. The section discusses the challenges of managing massive volumes and diverse types of data and explores solutions such as data warehouses, data lakes, and distributed computing frameworks.
Data Analysis Capabilities
This section examines the capabilities required for data analysis in Big Data Analytics. It discusses various techniques and methodologies, including statistical analysis, data mining, machine learning, and natural language processing. The section explores the challenges of analyzing large-scale datasets and emphasizes the importance of scalable algorithms and distributed computing platforms.
Data Visualization Capabilities
The focus of this section is on the capabilities related to data visualization in Big Data Analytics. It discusses the importance of effective data visualization techniques to communicate insights and facilitate decision-making. The section explores visualization tools, interactive dashboards, and visual storytelling approaches that enable users to understand and explore complex patterns and trends in big data.
Application of Big Data Analytics Capabilities
This section provides examples and case studies highlighting the application of Big Data Analytics capabilities across various industries, such as healthcare, finance, marketing, and manufacturing. It showcases how organizations have utilized these capabilities to drive innovation, optimize processes, and gain a competitive advantage.
Challenges and Future Directions
This section discusses the challenges and limitations associated with Big Data Analytics capabilities, such as data privacy, security, scalability, and interpretability. It also identifies research gaps and suggests future directions for advancing the capabilities of Big Data Analytics, including the integration of AI and cognitive computing, real-time analytics, and ethical considerations.
A Literature Review on Big Data Analytics
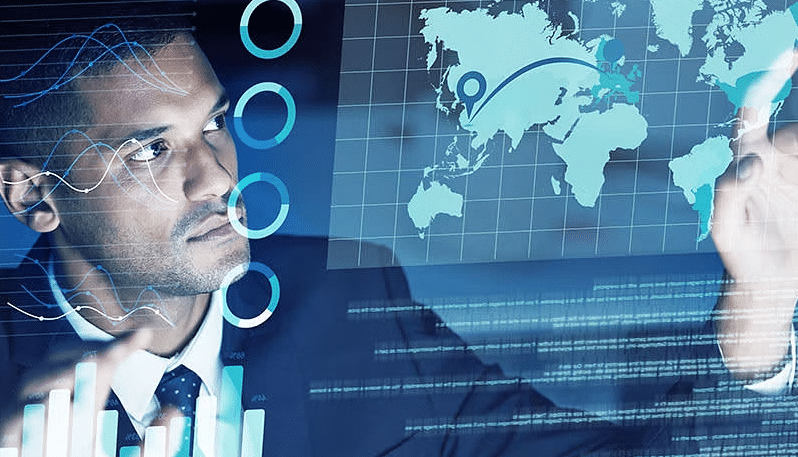
The introduction provides an overview of the importance of Big Data Analytics and its impact on organizations. It explains the motivation behind conducting a literature review and outlines the objectives and scope of the paper.
Definition and Concepts
This section discusses the definition of Big Data Analytics and presents key concepts, such as data volume, velocity, variety, and veracity. It explores the characteristics and unique challenges posed by big data, setting the foundation for understanding the subsequent sections.
Techniques and Methods
This section delves into the various techniques and methods employed in Big Data Analytics. It discusses descriptive, diagnostic, predictive, and prescriptive analytics techniques, along with data mining, machine learning, and statistical analysis approaches. The section highlights the strengths and limitations of each method and their applicability in different domains.
Challenges and Issues
Addressing the challenges associated with Big Data Analytics is crucial for successful implementation. This section examines the challenges related to data quality, privacy, security, scalability, and ethical considerations. It explores the complexities of integrating and analyzing diverse data sources, as well as the need for efficient data storage and processing infrastructure.
Applications and Use Cases
This section provides an overview of the applications and use cases of Big Data Analytics across various industries, including healthcare, finance, marketing, transportation, and social media. It highlights real-world examples where organizations have leveraged big data to gain insights, improve operational efficiency, enhance customer experiences, and drive innovation.
Research Gaps and Future
Directions Identifying research gaps and suggesting future directions is critical for advancing the field of Big Data Analytics. This section presents a synthesis of the gaps in the existing literature and proposes areas for further research, such as the development of privacy-preserving analytics techniques, real-time analytics, and interpretability of AI models.
Describe Data Analysis in a Research Paper
In a research paper, data analysis is described as the process of examining, organizing, and interpreting the collected data to derive meaningful insights and draw conclusions. It involves applying various statistical and analytical techniques to analyze the data and address research objectives or test research hypotheses.
When describing data analysis in a research paper, consider the following elements:
- Data Collection and Preparation: Provide a brief overview of the data collection methods and sources used in the study. Describe how the data was collected, whether through surveys, experiments, observations, interviews, or other means. Discuss any data preprocessing steps taken, such as data cleaning, transformation, or normalization, to ensure data quality and consistency.
- Analytical Approach: Explain the analytical approach employed to analyze the data. Discuss the specific statistical or analytical techniques used, such as descriptive statistics, inferential statistics, regression analysis, factor analysis, clustering, or thematic analysis, depending on the nature of the research questions or objectives. Justify why these techniques were selected and how they align with the research aims.
- Data Analysis Process: Describe the step-by-step process followed during data analysis. This may include detailing the software or tools used for analysis, the sequence of analyses performed, and any assumptions or conditions considered. Discuss any decisions made regarding data segmentation, aggregation, or filtering, as well as any statistical tests or models applied to the data.
- Results and Findings: Present the results of the data analysis in a clear and concise manner. Use tables, charts, graphs, or visualizations to illustrate the findings effectively. Discuss key patterns, trends, relationships, or statistical significance observed in the data. Ensure that the results are directly linked to the research questions or hypotheses stated earlier in the paper.
- Interpretation and Discussion: Interpret the findings within the context of the research objectives and existing literature. Analyze the implications of the results and discuss their significance. Identify any limitations or potential sources of bias that may affect the interpretation of the findings. Relate the results back to the research questions or hypotheses and offer explanations or insights based on the analysis.
- Conclusion: Summarize the key insights and conclusions derived from the data analysis. Address the research objectives or hypotheses and discuss how the findings contribute to the existing knowledge in the field. Highlight any practical implications or recommendations that emerge from the analysis.
By following these guidelines, you can effectively describe the data analysis process in your research paper, ensuring transparency, rigor, and clarity in presenting and interpreting the results.
Read More : The Seven V’s of Big Data Analytics